Ready to learn Data Science? Browse Data Science Training and Certification courses developed by industry thought leaders and Experfy in Harvard Innovation Lab.
Photo: Pixabay/www_slon_pics
‘Data Scientist’ might well be the sexiest job of the century. But hiring one is anything but that. Actually, it can be excruciatingly painful for companies. It’s an equally big deal for aspirants to bag that perfect offer in core data science, one which is not just a glossed-up, namesake role.
While machine learning is tough, training a human who can make machines learn can be tougher. One evolves through various incremental stages of expertise to become a productive data scientist.
For companies trying to identify one, it’s like finding a needle in the haystack. After years of hiring data scientists at Gramener, I’ve seen some conspicuously recurring patterns of skill gaps in the market. While there are hundreds of ways to fail an interview, these can be isolated into 4 broad paths.
The 4 pathways to Rejection
Given that only a handful from amongst thousands of applicants will crack that meaty machine learning position, it is helpful to understand where most people fail. For any aspiring data scientist or one looking to move up jobs, these are clear pitfalls to be avoided.
Becoming aware of one’s weakness is the sure and steady first step in fixing it.
Becoming a truly successful practitioner of data science involves picking up a specialised skillset. To illustrate nuances in the role in a light-hearted manner, we’ll explore parallels to each of these 4 points with the analogy of a person training to be a sniper; another cool, nuanced job calling for high skill levels.
Lets begin.. so, what are the 4 ways to fail a data scientist job interview?
1. Window dressing the CV with machine learning buzzwords
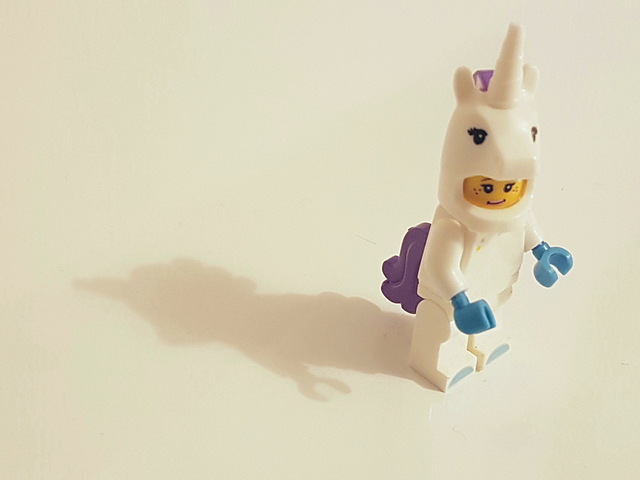
Photo by Inês Pimentel on Unsplash
As with any job, it may be tempting to tailor one’s resume by peppering it with industry jargons. And data science has no paucity for buzzwords. While this act of window-dressing does improve the chances of a CV getting picked by the automated scoring bots in HR, this can backfire rather quickly.
It’s not uncommon to find that advanced analytics skills claimed on paper actually translate to nothing more than basic familiarity with excel pivot tables, SQL queries or Google analytics. Even ignoring the time wasted, this poor tactic sets up candidates for big failure and a bigger demotivation.
For our aspiring sniper, this act equates to donning the garbs of a soldier and picking up a gun, without putting in any time needed in training to be one. As absurd as it sounds, its no fun for a sheep going to hunt in a wolf’s clothing.
2. Reducing model-building to just making library calls
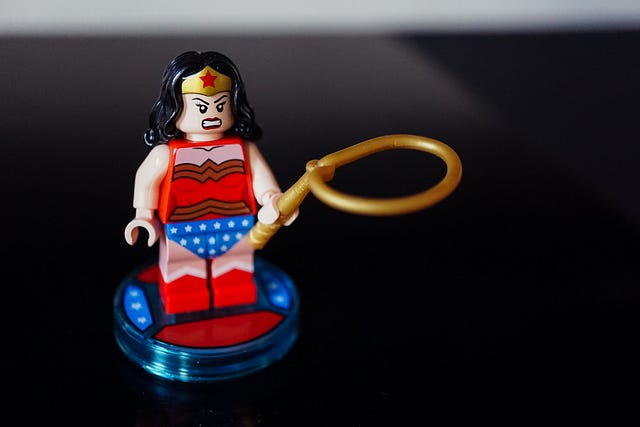
Photo by Zhen Hu on Unsplash
Many candidates who claim to know all about modelling, struggle greatly to explain beyond the model function calls and parameters. Even before asking what a technique like Random Forest does, a more important question is on why it is needed in the first place.
To be fair, a model is up and running with a single-line library call. But, machine learning is anything but it. One needs to understand, say, where logistic regression is more suitable than SVM. Or, when simple extrapolation is more powerful than forecasting techniques like ARIMA or Holt-Winters.
A good sniper needs to do a lot more than point and shoot. Actually, shooting is just 20% of the course in sniper school. One needs nuanced skills like patience, discipline and great observation to estimate target ranges from far.
3. Lacking the fundamentals essential for data analysis
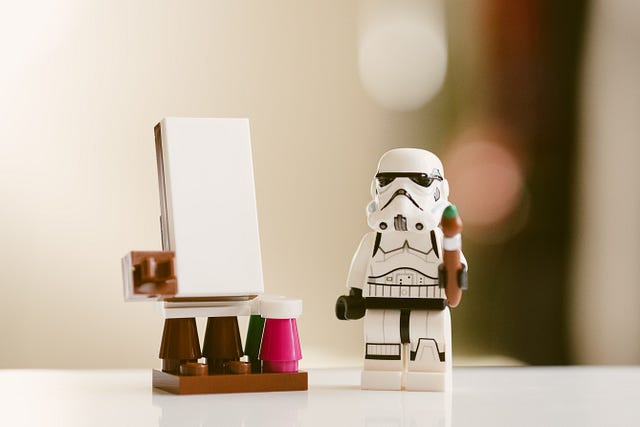
Photo by Daniel Cheung on Unsplash
While an intuitive understanding of machine learning techniques can serve as a strong plus for candidates, they often stop short at that. Investing in hands-on training to master more fundamental skills like statistics and exploratory data analysis are often overlooked.
Modelling accounts for just a small portion of the analytics lifecycle. In any successful ML project, over 50% of time is spent prior to that in data preparation, wrangling and approach. And almost 25% of time after, in model interpretation and recommendations.
Even as candidates flaunt 90% accuracy levels in projects, it’s a tragedy when they struggle to explain what a p-value is, and to see their diminished confidence in explaining why we need confidence intervals for models.
A firm grip on fundamentals is critical in all disciplines, and a sniper firstly needs to be a great infantryman. Of what use is excellent marksmanship, if one can’t fix a gun that jams or misfires in the midst of a battle?
4. Inability to apply analytics to solve business problems
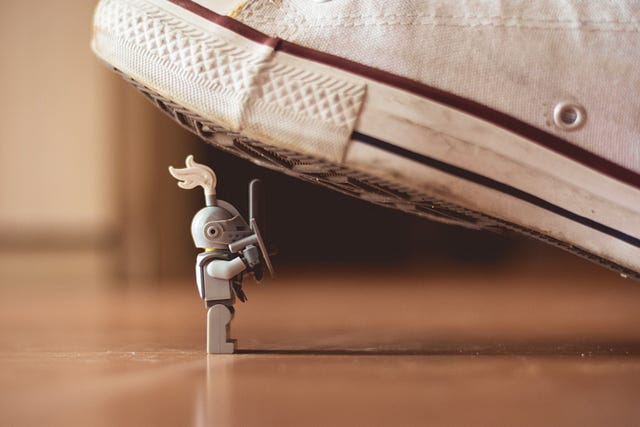
Photo by James Pond on Unsplash
It is clearly an uphill task mastering all aspects we’ve discussed so far. But we still miss a critical link in the chain, and this is where most interviews come to a screeching halt.
The ultimate mission for data scientists is to solve a business problem and not just analyse data or build a great model. This is the holy grail of data analytics. One needs to frame the right business questions, and evolve a sequence of steps to solve them. Even before loading any data into a tool.
When quizzed how a business can address their customer churn problem, it’s a conversation killer when candidates rush in with ideas of data analysis, or worse, toss around model names to predict churn. A better start is to probe on why customers sign up, the value they expect and what influences business.
Imagine an expert sniper who knows it all, but can’t conceal and camouflage in the ground or pick out the right targets to eliminate. Such an individual is truly a dangerous person, and the bigger risk is internal than for an enemy.
Wrap-up: In pursuit of data science
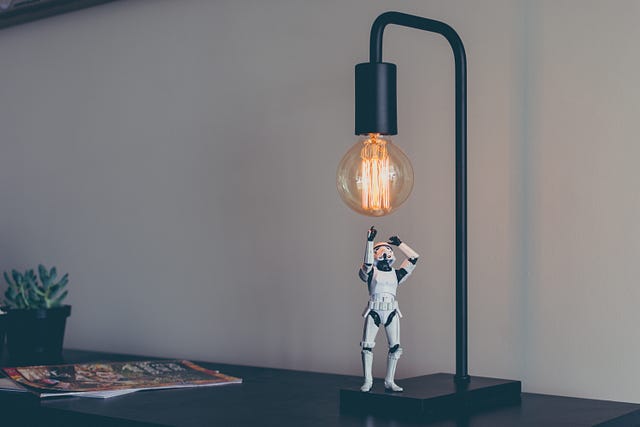
Photo by James Pond on Unsplash
In summary, one must adopt a disciplined pursuit towards data science by:
- Approaching a business problem by reframing the questions and evolving a sequence of steps to address the challenge,
- Applying the fundamentals skills in statistics and exploratory data analysis to get a feel for the data and iterate the analytics approach,
- Choosing a selection of analytics techniques or machine learning models, and then engineering and interpreting the results for business users
- And flaunting these skills with the right positioning of one’s expertise, for an ideal role fitment
So, good luck bridging the gaps and to create a dent in the analytics job marketplace
Plugging the 4 common failure points in Data science interviews