Has your CEO ever looked at a report you showed him and said the numbers look way off? Has a customer ever called out incorrect data in your product’s dashboards? I’m sure it hasn’t happened to you specifically, but perhaps you have a friend who had this problem?
In 2016, while I was leading a team at Gainsight, fondly called Gainsight on Gainsight (GonG), I became all too familiar with these issues. We were responsible for our customer data and analytics, including key reports reviewed weekly by our CEO and quarterly by our board of directors. Seemingly every Monday morning, I would wake up to a series of emails about errors in the data. It felt like every time we fixed a problem, we found three other things that went wrong. What’s worse, we weren’t catching the issues on our own. Instead, other people, including our very patient CEO, were alerting us about these issues. Recently, I talked to a CEO of another company who told me he used to go around the office and put sticky notes saying “this is wrong” on monitors displaying analytics with erroneous data.
At Gainsight, I had the privilege to work with hundreds of companies that were leading industries in their approach to customer data and deriving insights to improve business outcomes. But I saw firsthand that while many companies were striving to become data-driven, they were falling short. As they encountered pains and challenges similar to the ones I had, they lost faith in their data and ultimately made suboptimal decisions. A founder I met recently told me how persistent data issues in his company’s data and dashboards negatively impacted their culture and hindered their ambition to become a data-driven company.
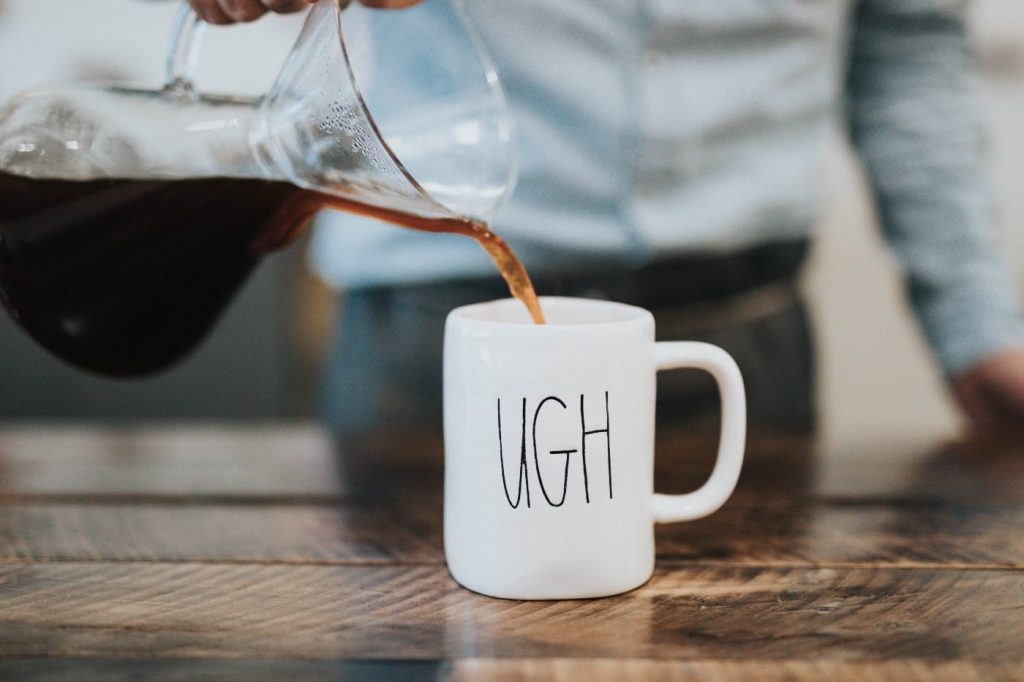
Introducing data downtime
I’ve come to call this problem “data downtime.” Data downtime refers to periods of time when your data is partial, erroneous, missing or otherwise inaccurate. It is highly costly for data-driven organizations today and affects almost every team, yet it is typically addressed on ad-hoc basis and in a reactive manner.
I call it downtime to harken back to the early days of the Internet. Back then, online applications were a nice-to-have, and if they were down for a while — it was not a big deal. You could afford downtime, since businesses were not overly reliant on them. We’re now two decades in, and online applications are mission-critical to almost every business. As a result, companies measure downtime meticulously and invest a lot of resources in avoiding service interruptions.
Similarly, companies are increasingly reliant on data to run their daily operations and make mission critical decisions. But we aren’t yet treating data downtime with the diligence it demands. While a handful of companies are putting SLAs in place to hold data teams accountable to accurate and reliable data, it is not the norm yet. In the coming years, I expect there will be increased scrutiny around data downtime, and increased focus on minimizing it.
Signs that data downtime is impacting your company
Data downtime may occur for a variety of reasons, including for example an unexpected change in schema or buggy code changes. It is usually challenging to catch this before internal or external customers do, and it is very time consuming to understand root cause and remediate it quickly.
Here are some signs that data downtime is affecting your company:
- Consumers of data, internal and external, are calling up with complaints about your data and are gradually losing trust. The customer might be thinking “Why am I the person spot checking data errors for this company?” Perhaps the CEO thinks: “If this chart is wrong, what other charts are wrong?”
- You are struggling to get adoption for data-driven decision making in your company, putting yourself at a disadvantage against your competitors. Folks might be saying “The data is broken anyway and we can’t trust it, so let’s just go with our intuition.”
- Your analytics/BI, data science, data engineering and product engineering teams are spending time on firefighting, debugging and fixing data issues rather than making progress on other priorities that can add material value to your customers. This can include time wasted on communication (“Which team in the organization is responsible for fixing this issue?”), accountability (“Who owns this pipeline or dashboard?”), troubleshooting (“Which specific table or field is corrupt?”), or efficiency (“What has already been done here? Am I repeating someone else’s work?”).
Tomasz Tunguz recently predicted that “Data Engineering is the new Customer Success.” In the same way that customer success was a nascent discipline and is now becoming a prominent function in every business, data engineering is expected to similarly grow. I couldn’t agree more and would add that the problem of data downtime spans many teams in data-driven organizations. As companies become more data-driven and sophisticated in their use of data, along with business functions increasingly becoming heavier consumers of data, I expect the problem of data downtime to amplify and grow in importance. I also expect better solutions will emerge to help teams across the industry mitigate data downtime. In a future post, I’ll describe how we attempted to solve this problem at Gainsight — and the benefits companies achieve when they regain trust and confidence in their data.
This article was published on Monte Carlo.