The article explains two high-end Cloud data storage technologies, namely, Data Lake and Data Warehouse. The purpose of this post is to compare the differences and capabilities of Data Lakes and Data Warehouses to help businesses make the right decision on selecting the right technology.
Data is the most valuable asset for companies because it facilitates decision making, so its management is the main responsibility. Companies need to understand the real value of data management technology to sustain an ever-changing economy. Since the volume of data is bloating at a faster rate, the organizations must decide as to what data storage technique they would prefer for storing big data.
In today’s modern technology landscape, Data Lake and data warehousing are unquestionably the two widely used Cloud data storage technologies for storing big data. Although it is a variety of techniques that serve completely different purposes than some overlap, it is sometimes used interchangeably.
Let’s understand the data lake and data warehouse technologies to understand the purpose, features, and benefits.
What is a Data Lake?
Data Lake, a centralized repository, allows for storing high volumes of structured, semi-structured, and unstructured data. It stores data in its native formats. Unlike traditional relational databases that process the raw data before storing it, data lakes can store raw data without the need without processing or transforming it.
Data in data lakes is stored at a relatively faster speed and is available quickly for access. In short, the data lake stores all kinds of data from all sources, irrespective of its format.
What is a Data Warehouse?
Data warehouse stores data after it is being extracted, processed, and stored into files and folders. Cloud Data warehousing solutions for storing structured data from one or more resources. When data is stored in an organized format into files and folders, it is easily available and helps to take strategic, data-driven decisions.
Data warehouses store data in quantitative metrics with defined attributes. It is a combination of high-end technologies that allows for strategic data usage.
Comparison between Data Lake and Data Warehouse Technologies
Let’s understand some major differences between the two modern data storage and management technologies based on essential parameters.
Storage
Data Lakes stores raw data in all structures and sources, while data warehouse stores only structured data consist of quantitative metrics.
Data Capturing
Data Lakes captures data in original formats that include structured, semi-structured, and unstructured data across disparate resources. On the contrary, a data warehouse captures structured information and later organize its schemas.
Data Processing
Data lakes use ELT (Extract Load Transform) process for data processing, while data warehouses utilize the ETL (Extract Transform Load) process, which is relatively traditional.
History
Data Lake uses relatively new big data technologies, whereas the Cloud Data warehousing technique has been around for decades.
Cost
Storing data in Data Lakes is relatively affordable as they are designed for low-cost storage. On the other hand, Data Warehouses are costly, particularly when you need to store large chunks of data.
Users
Data Lake is an optimal option for users who are engaged in deep analysis, such as data analysts, data scientists. It’s useful for users who need access to advanced analytical tools with statistical analysis and predictive modeling capabilities. Data warehouse, due to its support for structured data and ease of use, is more preferred among IT and business users.
Position of Schema
Data lakes technology usually defines schema after data is stored in the repositories. It simplifies capturing data and provides more agility. On the contrary, the schema in the data warehouse is defined before storing data.
Agility
Data lakes, because of lack of structure, offer more agility, thus simplifies it easier for data experts to configure/reconfigure queries, data models, and applications. Data warehouses are highly structured data repositories with a definite configuration. It is not as agile as a data lake, but changing the structure is time-consuming.
Processing Times
Data lakes provide faster access to data even before it is processed, transformed and cleansed. It allows users to make data-driven decisions much faster. Data warehouses provide data insights when it is processed and stored into more defined formats.
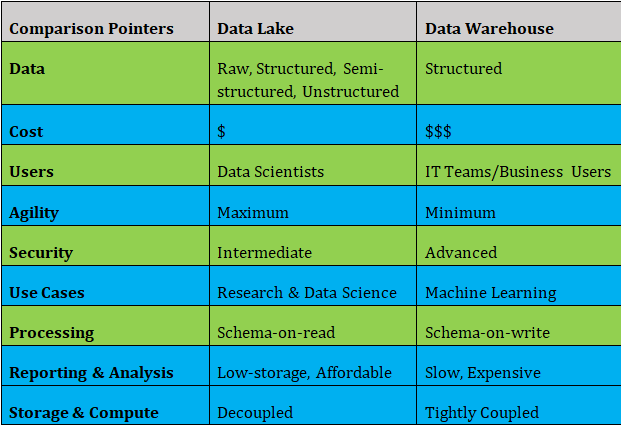
Would You Go with Data Lake OR Data Warehouse?
A data lake is a new technology, but there are several challenges associated with this data storage approach. This technology may not resolve all your data-related problems and may ignite them. Since data lakes allow storing almost everything, it seems quite unfeasible as users will find it difficult to fetch a value from data.
As data lakes support all kinds of formats across disparate sources, users experience higher latency. Since data scientists and analysts utilize it, a little lag in information can affect the overall analysis process. Lack of data prioritization in data lakes can obstruct the analytical process.
However, the decision or choosing data lakes or data warehouses depends truly on the business-specific requirements and the nature of the industry.
Education Industry: Since data lakes offer agility, it can be an ideal data storage option for educational institutions.
Healthcare Industry: Due to the unstructured nature of data such as patient’s medical history, clinical reports, physician notes, etc., data lakes are more feasible for the healthcare industry.
Finance Industry: Data warehouses can be a better option for banking and financial institutions as it facilitates an organized data storage format with high accessibility.
Future of Data Warehousing Technique is Bright
While designing machine learning models, companies usually spend the majority of time preparing data. Building machine learning programs demand up-to-minute information; data warehouses will become essential for Artificial Intelligence and ML models.
Data warehouses make data preparation easy due to its integrated transformational capabilities. Data warehouse companies are working consistently to improve the Cloud experience for customers and making it flexible, affordable for end-users.