New regulations impose obligations, including post-market monitoring, on all companies using “high-risk” AI in the EU
Last week, a draft of the EU’s highly anticipated Regulation on A European Approach For Artificial Intelligence was leaked. The official version is expected this week.
Although as non-lawyers, we cannot offer a legal analysis of the regulation, we can say that the draft makes clear that the EU is preparing to take a robust approach to regulating AI, from ensuring good data sets, to proper testing and training of the system, required registration in a newly created EU database, mandatory post-market monitoring, and penalties for those who fail to comply.
The regulation has broad implications. Although most of the provisions are limited to “high risk” AI systems, “high risk” seems to be defined quite broadly. The draft directly lists some “high risk” AI use-cases such as employee / candidate assessment, determining creditworthiness and operating essential public infrastructure. Additionally, the draft declares that the parameters defining “high risk AI” would be dynamic, and that the commission is empowered to include additional use-cases under these definitions according to the severity and probability of causing harm.
Time will tell just how broadly this regulation is applied and how many different types of AI systems are covered. However, as the term “high risk” is broadly defined, and the regulation encourages providers of non-high-risk AI to comply as well, forward-thinking companies should start planning now how they will comply with this new, sweeping regulation.
So, what should you do to prepare?
1. Time to change your thinking
Throughout the draft, “AI systems” and not “models” is the prevailing term. This shift from “model” to “system” may be challenging for data scientists as traditionally, the “model” has been the end goal of the research project. Additionally, many of the tools used by data scientists are model centric. That might work for the research phase, but production systems typically contain more than the one model (and many other pieces besides the model), and it’s the entire AI system that will need to be registered (yes, all high-risk AI systems will be registered in an EU database). It’s the entire AI system that will need to pass conformity tests.
2. Get organized!
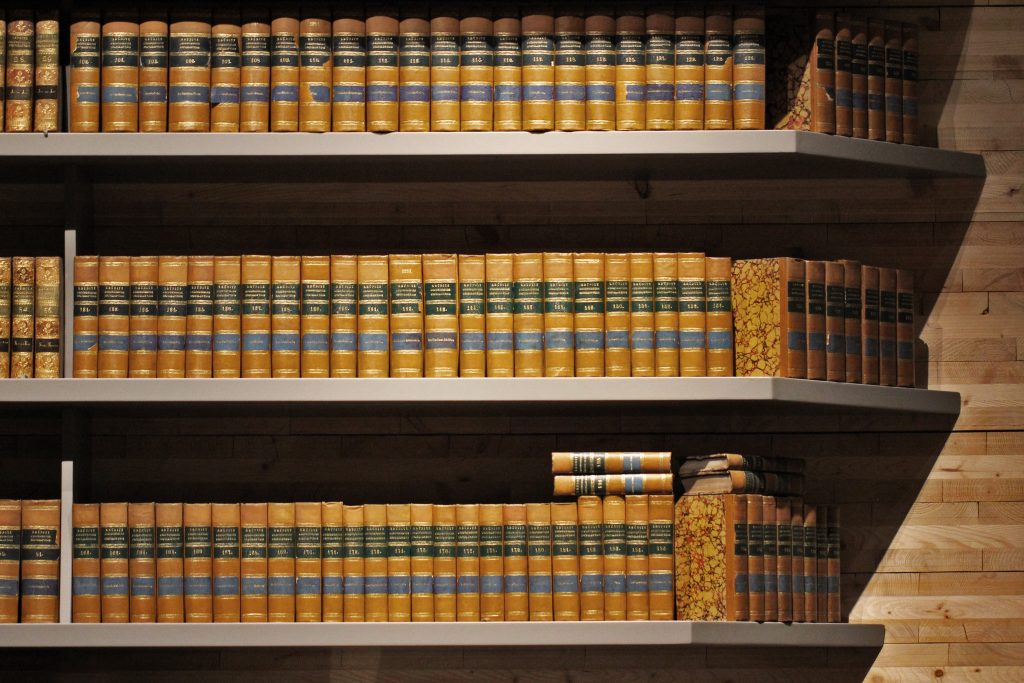
The draft makes clear that your AI system must be organized, transparent, and documented. Specifically, you must:
- Save the datasets on which you train and test each model version
- Create clear technical documentation for your system, which can trace back every decision you made in building it
- Be transparent and inform your users about considerations when using your AI system
Fortunately, an array of tools already exist to help with documentation and record keeping around your datasets, models and system design. MLFlow and other ML platforms enable you to manage experiments, register models and save training data, while end-user communication tools are already common in every organization.
3. Ensure human oversight of your AI system
A key point of the regulation is that AI systems must have human oversight. Instead of just being “in the loop”, a person must be on point to be alerted when something unexpected happens. This person must be able to understand what is happening and have the ability to override the system.
Already today, in mature data-driven processes, such as fraud detection and risk assessment, human analysts supplement models and forecasts. We predict that in many other sectors, and certainly in “high risk” AI driven processes, such analysts will be trained and equipped with tools for a better human-machine interface.
4. Institute a smart go/no-go mechanism to avoid bias when updating your AI system
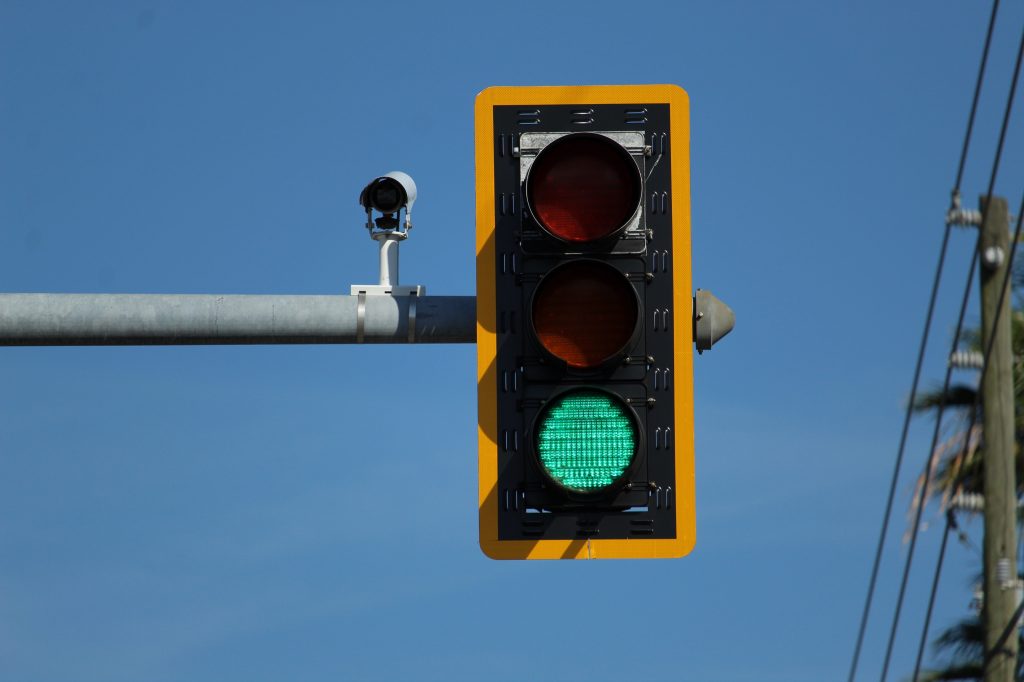
The quality of your data matters. The draft requires you to ensure that your training and test data does not contain low-quality data or biases.
We draw two implications from these data quality/bias related provisions. First, you must look beyond inputs and outputs of models. Leverage metadata (e.g., race, gender) and business dimensions (e.g., geolocation) to assess your data and the entire system’s behavior in subpopulations to ensure appropriate statistical representation and avoid bias. Second, teams will need to establish a robust, automated process to validate that there’s no bias whenever new versions are released (and block releases when biases exist).
5. Implement an end to end AI monitoring solution
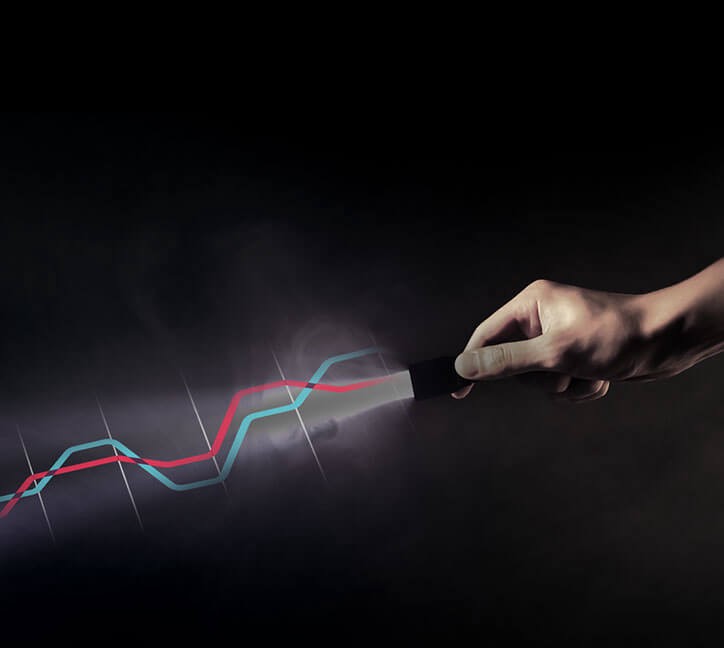
The regulation is specific in its requirements for a post-market monitoring system. According to section 83 in the draft:
In order to ensure that experience from the use of high-risk AI systems they design and develop is taken into account for improving the development process or take any possible corrective action in a timely manner, all providers should have a post-market monitoring system in place.
If you’ve been following our writing, you know that we believe that a comprehensive monitoring strategy can make a real difference for teams, aiming to turn their AI research investments to scalable business operations.
The draft specifically discusses “AI systems which continue to learn” in production. This is an acknowledgment that potential risks of AI systems emerge over time, and cannot be pre-mitigated when these systems are initially launched. Another strong argument for monitoring being a critical enabler for safe, and reliable continuous evolution in AI environments.
In conclusion
The EU is leading the charge to regulate and manage the vast emerging AI market. However, we are confident that we will see similar regulations from other governments in the near future. The implications for the entire AI industry are huge, and it is best to get out in front of and prepare for these regulations now by implementing the right tools and processes.
Are you on a team building or operating an AI system that could be covered under these new regulations? Get in touch. We would love to hear how you are thinking about navigating this new regulatory landscape.
Thanks to Yotam Oren for collaborating with me on this. Originally posted on blog.monalabs.io.