Ready to learn Artificial Intelligence? Browse courses like Uncertain Knowledge and Reasoning in Artificial Intelligence developed by industry thought leaders and Experfy in Harvard Innovation Lab.
When big potential doesn’t translate to business benefits
So, you’ve heard the dazzling sales pitch on deep learning and are wondering whether it actually works in production. The top question companies have is on whether the promised land of perennial business benefits is a reality.
In a previous article, we saw a simple business introduction to deep learning, a technology that seems to have a swashbuckling solution to every problem.
But, what happens when the rubber hits the road?
A good gauge of an innovation’s maturity level is by understanding how it fares on the ground, long past the sales pitches. We’ve been studying advances in deep learning and translating them into specific projects that map to client problems.
Here are five reasons deep learning projects come to a screeching halt, even as they get started with the best of intentions:
1. Expectations bordering science fiction
Yes, AI is fast becoming a reality with self-driving cars, drones delivering pizzas and machines reading brain signals. But, many of these are still in research labs and work only under carefully curated scenarios.
There’s a thin line of separation between what’s production ready and where it’s still a stretch of imagination. Businesses often misread this and amidst the euphoria to solve ambitious challenges, teams wade deep onto the other side.
This is where AI disenchantment can happen, prompting businesses to turn over-cautious and take many steps back. With some due diligence, the deep learning use cases that are business-ready must be identified. One can be ambitious and push boundaries, but the key is to under-promise and over-deliver.
2. Lack of data to satiate the giant’s appetite
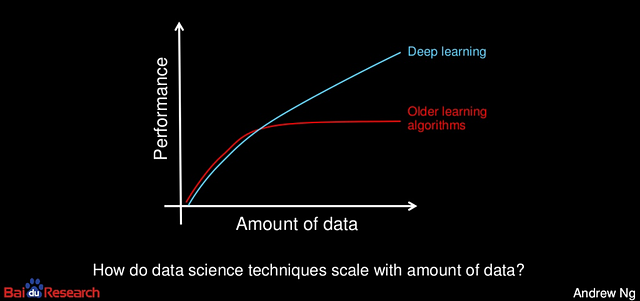
Performance of analytics techniques with data volume, by Andrew Ng
Analytics delivers magic because of data, and not in spite of its absence. No, deep learning doesn’t solve this festering challenge of data unavailability. If anything, deep learning’s appetite for data is all the more insatiable.
To setup a simple, facial recognition-based attendance system, training data needed is the mugshots of employees. These pictures may be recorded live, or submitted with some variations of features (orientations, glasses, facial hair, lightings..). Usually, such data gathering can quickly turn into a mini project.
Project sponsors often assume availability or the ease of collection of such data. After the best of efforts, they end up with just partial data that delivers moderate accuracy. This slight shortfall can mean the difference between production-grade solutions and just an attractive research prototype.
3. Lots of training data, but none labelled
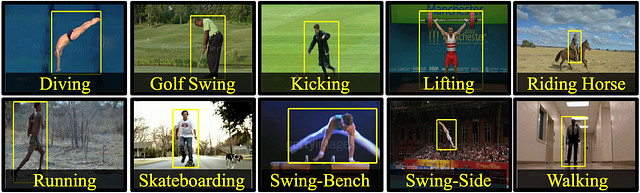
Labeled dataset on Sports actions from UCF
When a curated database of a million data points is at one’s disposal, is that sufficient for deep learning to unfurl the magic? Well, not so fast. The training data needs painstaking labelling to get the model to learn. This is often overlooked.
Algorithms need boxes drawn around pictures to learn spotting the people. Faces need labeling with a name, emotions must be tagged, speaker’s voices called out and even a table of numbers described with detailed metadata.
‘Wow, that’s a lot of work’, one might say. But that’s the tradeoff in teaching deep learning models, if we are to absolve ourselves of the even more painful process of feature extraction (the job of deciding if eyes or nose differentiate the faces).
4. When the cost-benefit tradeoff doesn’t stack up
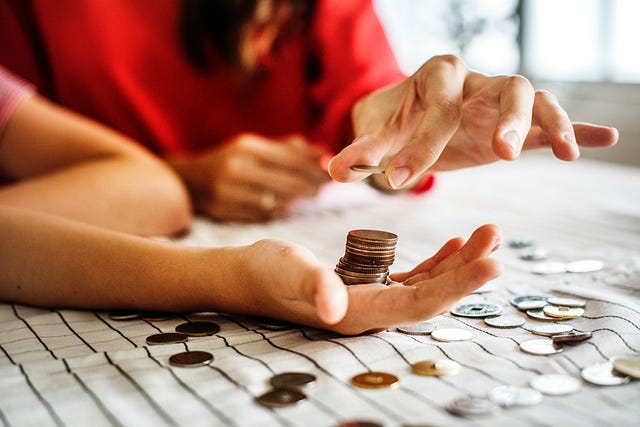
Photo by rawpixel on Unsplash
The efforts to gather and label data, combined with GPU-grade computing can prove costly. Add in ongoing efforts to maintain production models with labeling, training, and tweaking, and the total cost of ownership shoots up.
In some cases, it’s a late realization to find that staffing people for manual inspection and classification can be cheaper than going about this rigmarole. Talk about large volumes and scalability, then deep learning again starts making sense. But, not all businesses have this need as a priority, while getting started.
With research in deep learning progressing steadily, this is changing by the day. Hence, it’s critical to examine the deep learning total cost of ownership, early on. At times, it may be wise to defer investments until the cost economics become favorable.
5. When insights are so smart that people get creeped out
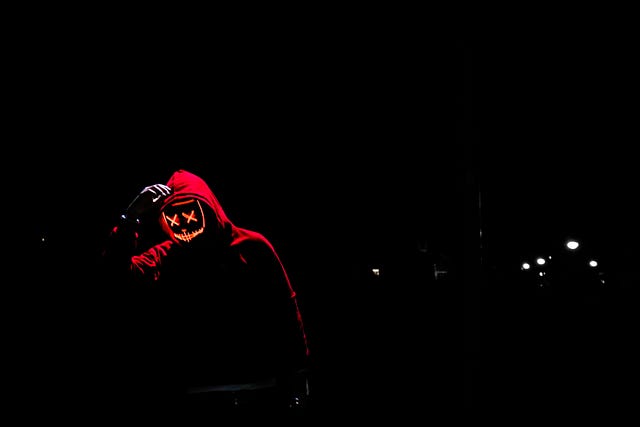
Photo by sebastiaan stam on Unsplash
This concern falls on the opposite side of the spectrum. There are use-cases which turn out to be sweet-spots for deep learning where data availability and business needs are ripe for use. The stars are aligned, and the model turns prophetic!
The challenge here is that the model knows way too much, even before people verbalize the need for it. And that’s when it crosses the creepiness zone. It may be tempting to cross-sell products before the need is felt, or detect deeper employee disconnects by tracking intranet chatter.
But these stoke questions of ethical dilemma or cast doubt on data privacy with customers or employees. When in doubt whether a use-case can alienate the target audience, companies must give it a pass, in spite of the potential at hand. Remember, with great powers come greater responsibilities.
Summary
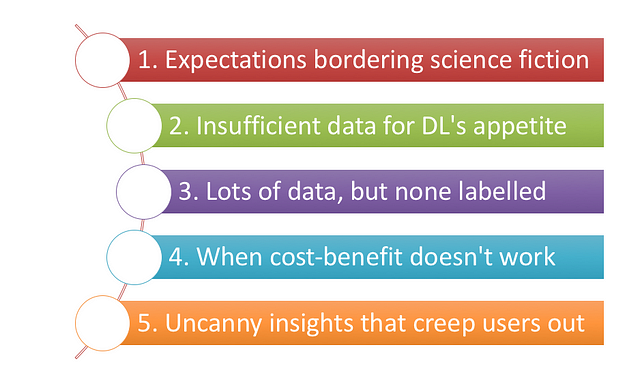
5 Reasons why Deep learning projects fizzle out
It’s heady days for deep learning with the stellar advances and infinite promises. But, to translate this unbridled power into business benefits on the ground, one must watch out for these five pitfalls.
Ensure availability of data, feasibility of labeling them for training, and validate the total cost of ownership for business. Finally, scope out the right use-cases that excite and empower users without creeping them out.
You may wonder when deep learning must be used vis-a-vis other techniques. Always start with simple analysis, then probe deeper with statistics, and apply machine learning only when relevant. When all these fall short, and the ground is ripe for some alternate, expert toolsets, dial in deep learning.