About the art of ranking and doubling-down on the next best AI Starship.
With a sea full of Artificial Intelligence (AI) Project ideas, it is hard to figure out where to start fishing. Companies are becoming increasingly aware of the benefits of AI. The private equity sector invested more than 50bn $ in AI startups in the last six years. Where should you start your AI Journey? How do you rank different AI Projects? What AI Projects should you focus on depending on the level of AI Maturity of your business?
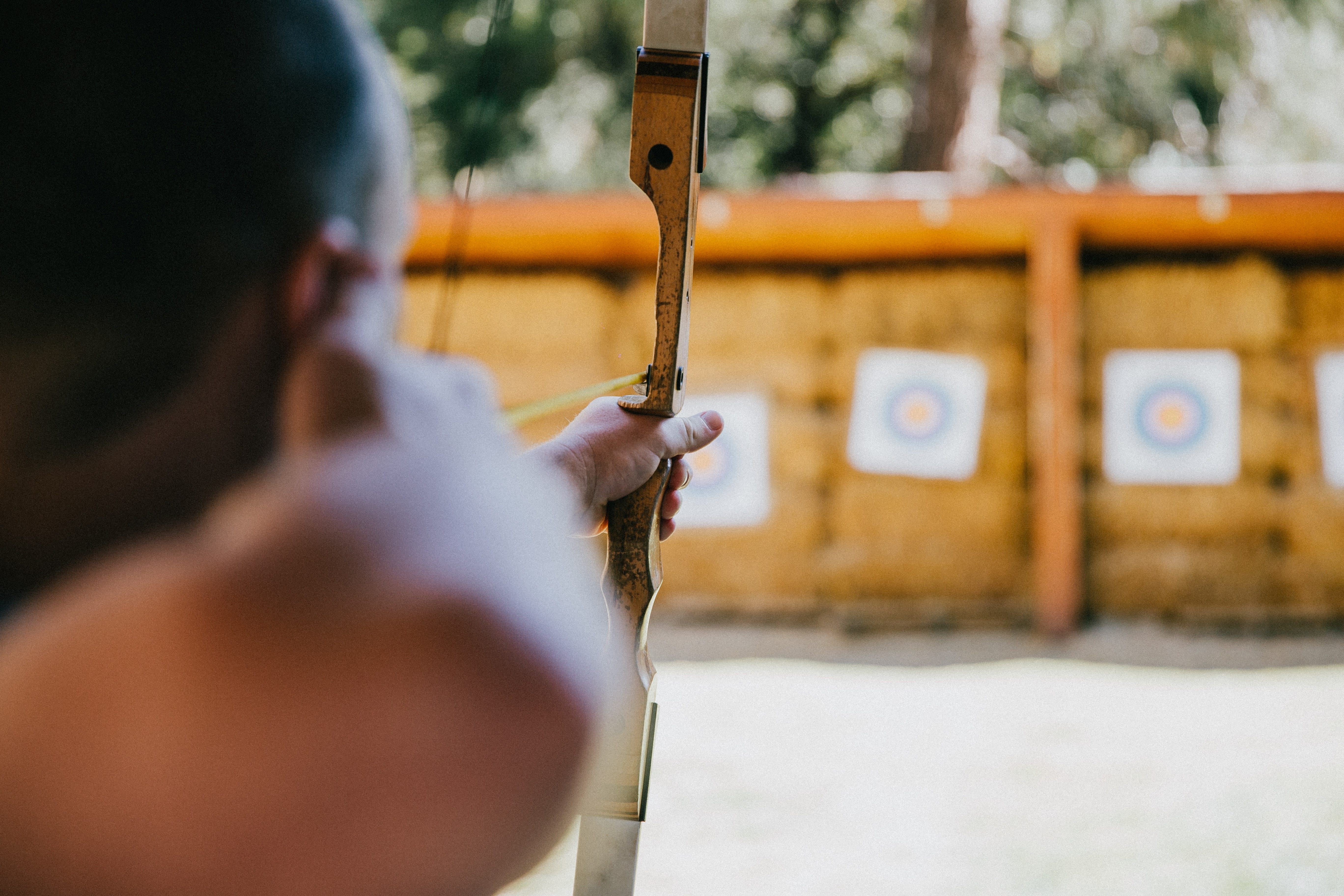
Photo by Vince Fleming on Unsplash
This article explains how to approach evaluating and ranking different AI Projects. Every organization has limited resources which they need to allocate to maximize impact. Depending on your position along the AI Maturity Journey, the trade-offs between impact and risks of AI Projects become more important. The insights are derived through discussions with industry-experts at Porsche AG, the Silicon Valley startup Xcalar, and my own experience.
Let’s read on.
Using the Impact-Risk AI Project Matrix
The main dimensions to evaluate AI Projects are impact and risk. Impact defines how valuable the AI Project is, risk how likely it is to fail. AI Projects can be clustered in four groups depending on their estimated level of impact and risk.
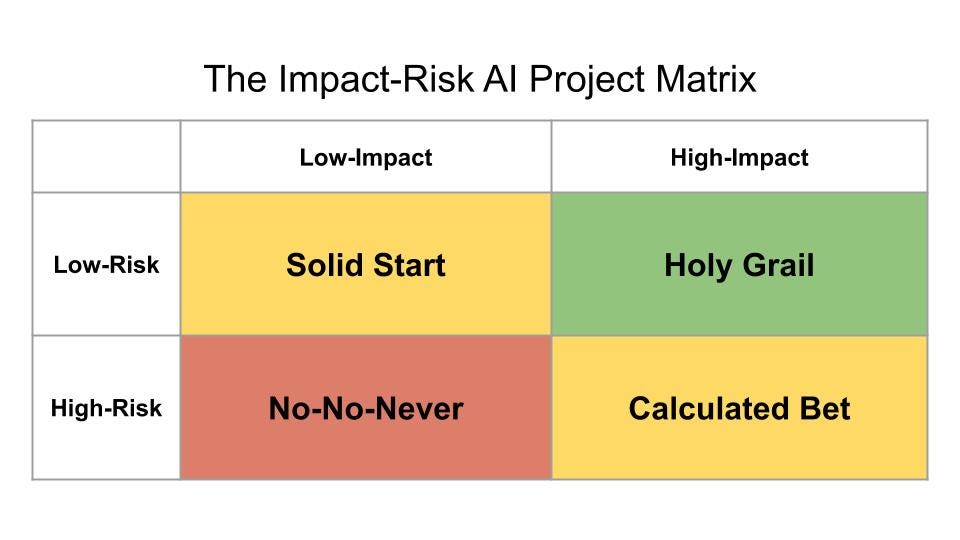
AI Projects that are low-impact, low-risk fall into the “Solid Start” category. These projects can be helpful to start your AI Journey but are not relevant once your company reaches an advanced level of AI Maturity.
AI Projects that are high-impact, low-risk, are the “Holy Grail” projects to search for. These projects are both likely to succeed and have a major impact on business objectives. If you discover these projects, hold them dearly and focus your efforts on making them work.

The Holy Grail AI Projects everyone is looking for. Source: Wikimedia
AI Projects that are high-impact, high-risk are considered “Calculated Bets”. It is uncertain that the project succeeds, but if your talented engineers find a way to make it work, the project promises major payoffs in return.
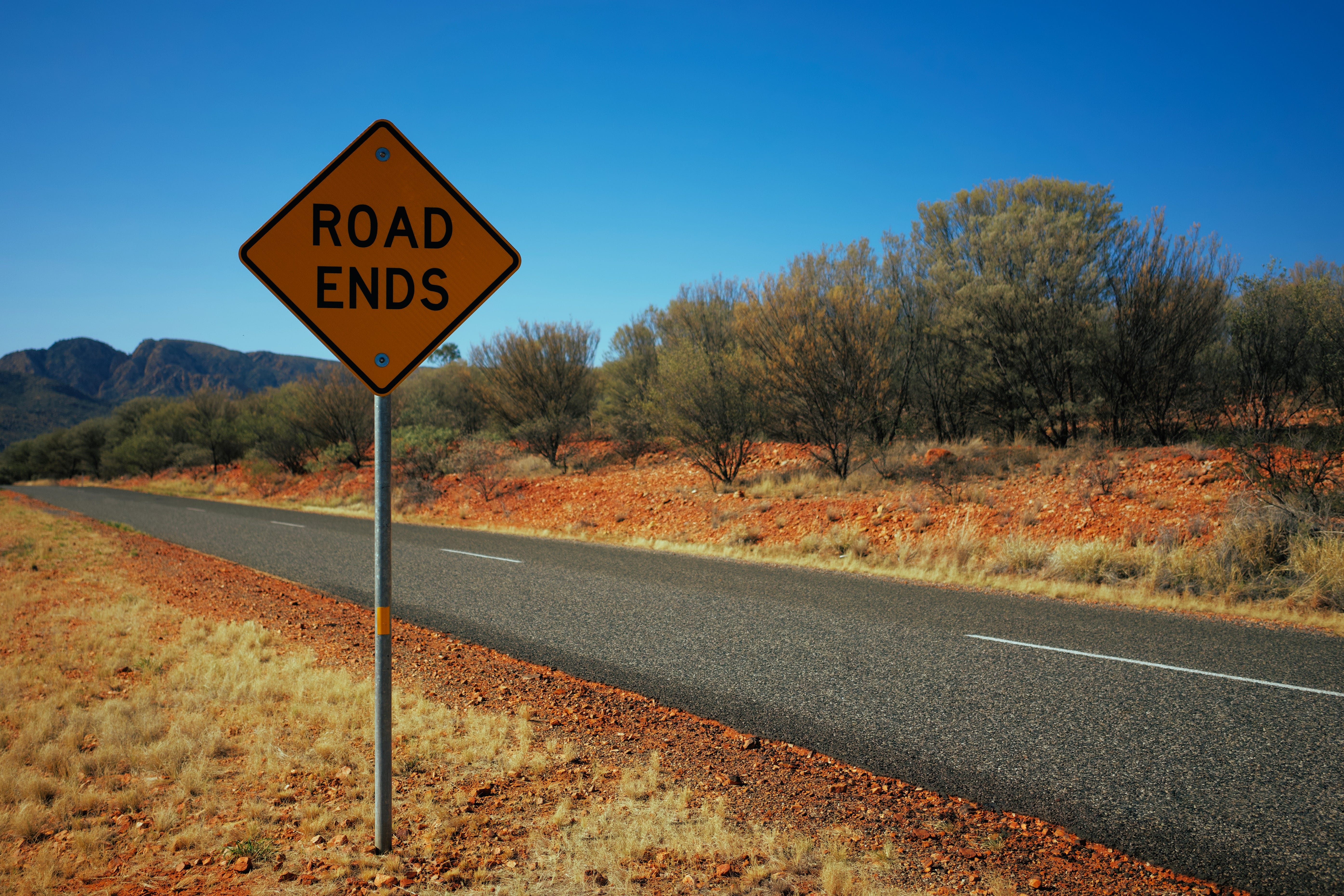
Photo by Lubo Minar on Unsplash
Now, how do you assess the impact and risk of AI Projects to rank them? The following sections dive deeper into the two criteria.
Impact
An AI Project is meaningful if it impacts the business objectives. Every company has objectives, KPIs, or OKRs, by which it steers the business. Some companies even employ a successful AI Strategy. AI Projects should support the AI Strategy and thus the business objectives.
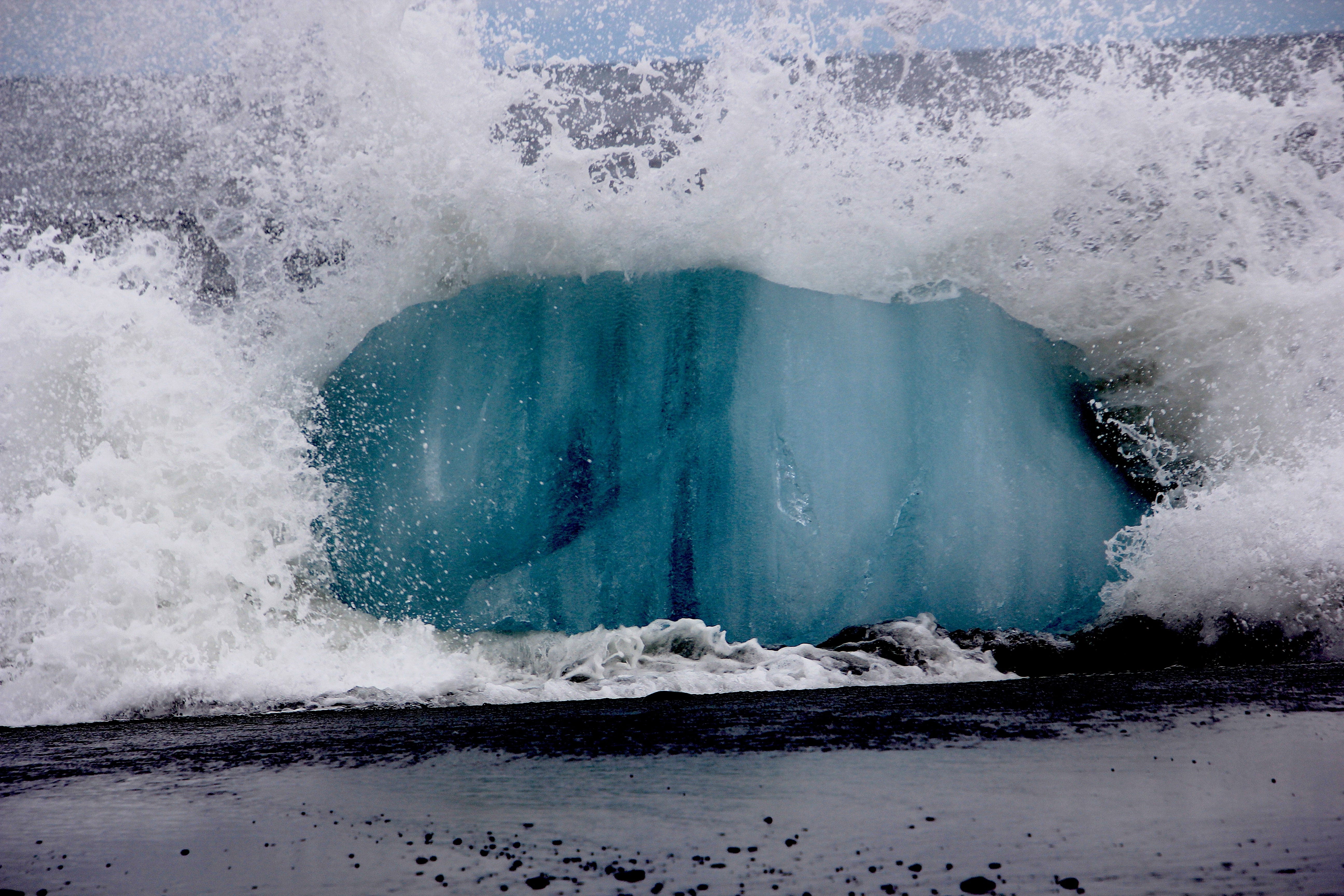
Photo by James Toose on Unsplash
Is all impact created equal?
Tobias Grosse-Puppendahl, ex-Microsoft Researcher and now Innovation Manager at Porsche AG, differentiates three categories of value delivered by AI: business, customer, and knowledge value. Business value impacts the financials of a company directly through new products or decreased costs. Customer value focuses on driving user engagement. Knowledge value enables employees to adopt new skills or be able to make more informed decisions in the future (e.g. by collecting valuable data).
Depending on your company situation, focus on connecting impact to these three areas.
How do you calculate the potential impact of AI Projects?
The difference between low- and high-impact AI Projects is the size of the addressable audience and the financial opportunity. You can define impact as
Impact = Addressable Audience * Opportunity .
Addressable Audience is defined by how many users, employees, or produced goods are affected by a change. Opportunity addresses the expected change in financial or KPI data. This includes the costs to deliver the project. You can estimate the opportunity by comparing your situation to the outcomes of competitors or your own predictions. Multiply these two factors together and you have a measure of impact.
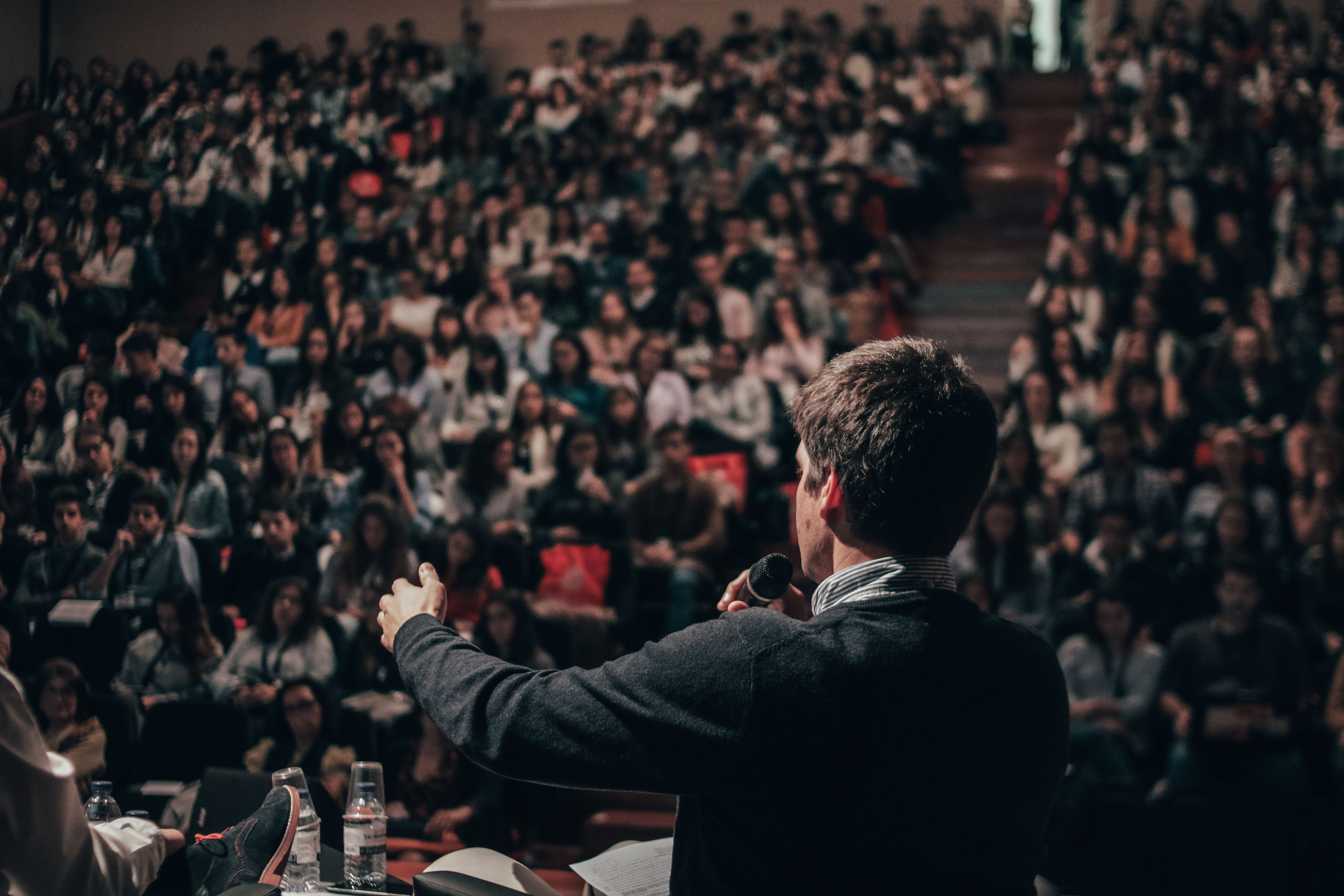
Photo by Miguel Henriques on Unsplash
For example, let’s assume you have 1’000 users who spend an average of 10$ on your website every month. We expect that a recommendation system will increase profit by 5%. As a comparison, McKinsey states that the recommendation engine already generates more than 35% of Amazon’s revenue. Since we are not Amazon, a 5% increase seems reasonable. This equals an impact of (1’000 * 10$) * 0.05 = 500$ in additional profit every month. Glad that we have our business basics on lock! If we calculate the impact for all projects, we can group them into high- or low-impact categories already.
Risk
Risk is defined by the chance that the project will not succeed given time, costs, and quality restraints. The riskier a project is, the more likely it is to fail.
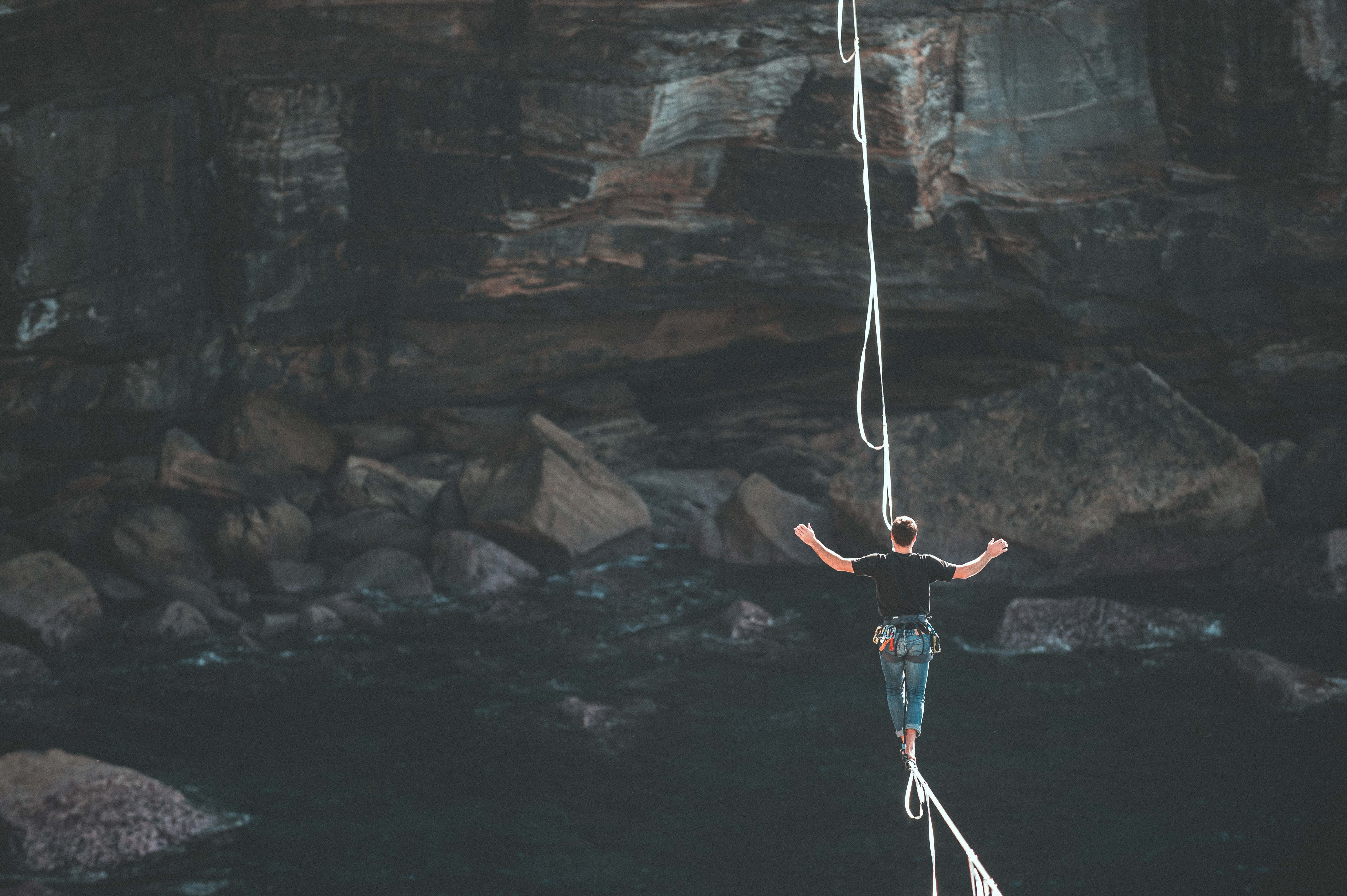
Photo by Loic Leray on Unsplash
What factors define the riskiness of an AI Project?
Every AI Project feeds off the holy trinity of AI through data, algorithms, and infrastructure. If data is not available, the algorithms are new territory, and you don’t have a fitting infrastructure, it becomes really hard to implement an AI Project. Hence, it becomes riskier.
OpenAI’s Josh Tobin states in the Full Stack Deep Learning course that the main factors that define the feasibility of an AI Project are data availability, accuracy requirements, and the complexity of the problem. Generally speaking, data labeling and accuracy requirements are often the main cost factors (Week 2, Min 29). The more labeled data is needed and the higher the accuracy requirements are, the more costly your project will become. Creating self-driving cars requires high accuracy and is thus really expensive. On the other hand, creating a basic online recommender generally has limited data labeling and accuracy requirements and is thus cheaper and less risky.
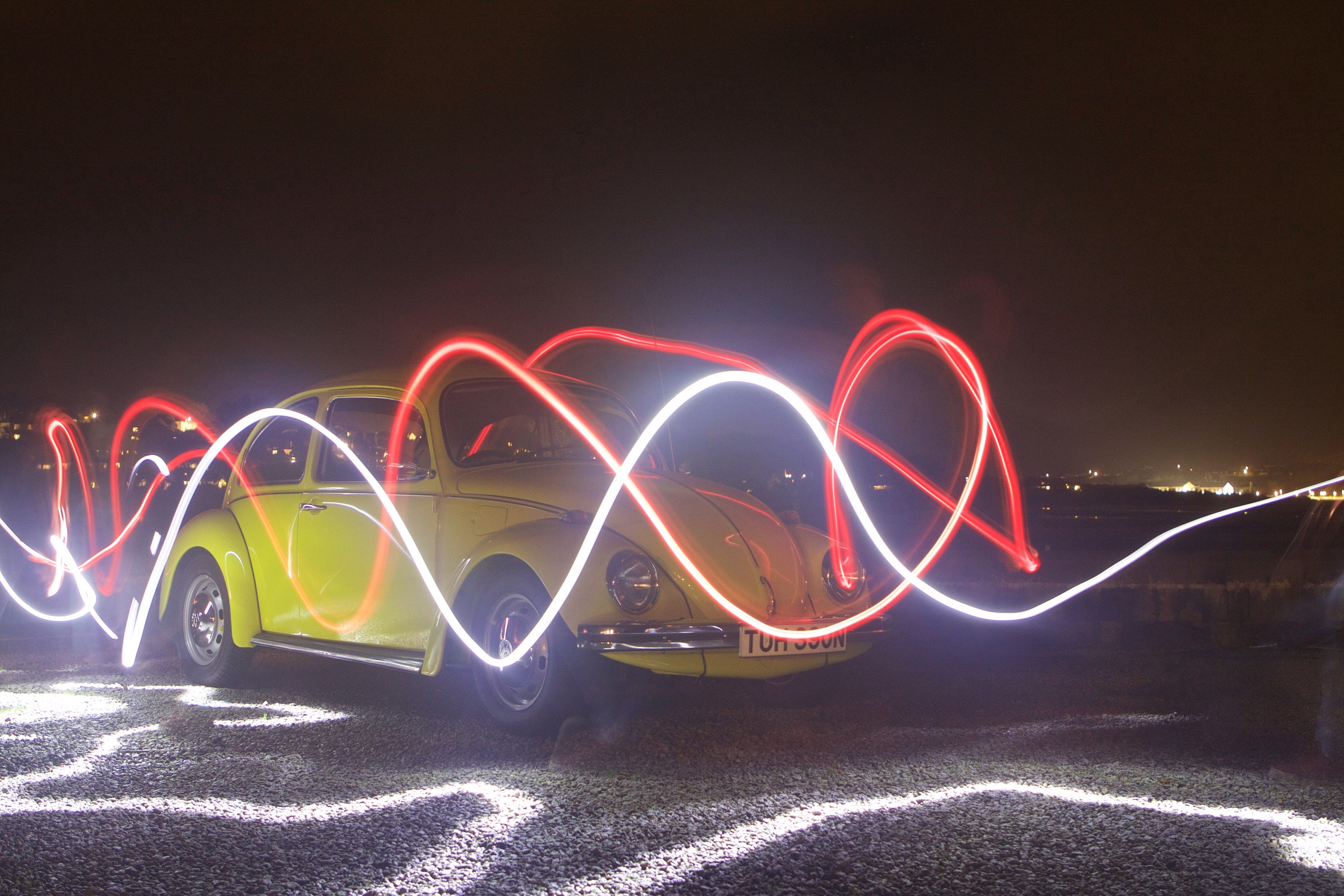
Photo by Matthew Szlichta on Unsplash
Rebecca Ye, ex-Google PM and now Co-Founder and COO of enterprise startup XCalar, prioritizes the ties to a business outcome as the main risk factor of every AI Project. Rebecca sees that many large corporations are just starting out with AI. To have sustainable investment and implementation of AI, pull from business units is paramount. AI Projects should understand that they need to meet business goals.
Now that we have a list of risk factors for AI Projects, let’s standardize AI Project risk assessment.
What tool can you use to define the riskiness of an AI Project?
While the risk of an AI Project is not as handily calculated as the impact, the AI Project Canvas serves as a good proxy to evaluate riskiness. The AI Project Canvas examines the value proposition, data, customer, and financial perspective of the project. Thus, it answers the main factors that Josh and Rebecca mention above.

The more categories you can answer in the AI Project Canvas, the lower the risk of your AI Project is. If you have a clear value definition, data is readily available, you know how to ship the product to your customer, and you have an eye on project finances, then you are on a path to success. Use the number of convincingly answered categories to separate projects into high- and low-risk AI projects.
As the last step, now that we rank our projects by impact and risk, which projects should we focus on depending on the level of AI Maturity?
Placing your bets depending on your AI Maturity Level
Andrew Ng, former Google and Baidu Brain Lead and now CEO of landing.ai, recommends companies to simply get started with AI. In the AI Transformation Playbook, starting with AI Projects is the very first bullet point. In the online course AI for Everyone, he further explains that starting with AI is more important than having an AI Strategy. We’d like to go a step further and propose to look at the impact and risk of AI Projects before getting started with AI.

Typically, companies embarking on the Journey to AI Maturity find themselves in one of three maturity levels: Beginner, Advanced, Professional. Beginner companies don’t have a working infrastructure, no overview of their data capabilities, and just hired the first few data employees. Advanced AI organization have delivered the first AI projects successfully. Professional AI companies have established AI as one of their main competitive advantages and reap benefits from a working infrastructure and highly-skilled engineers.
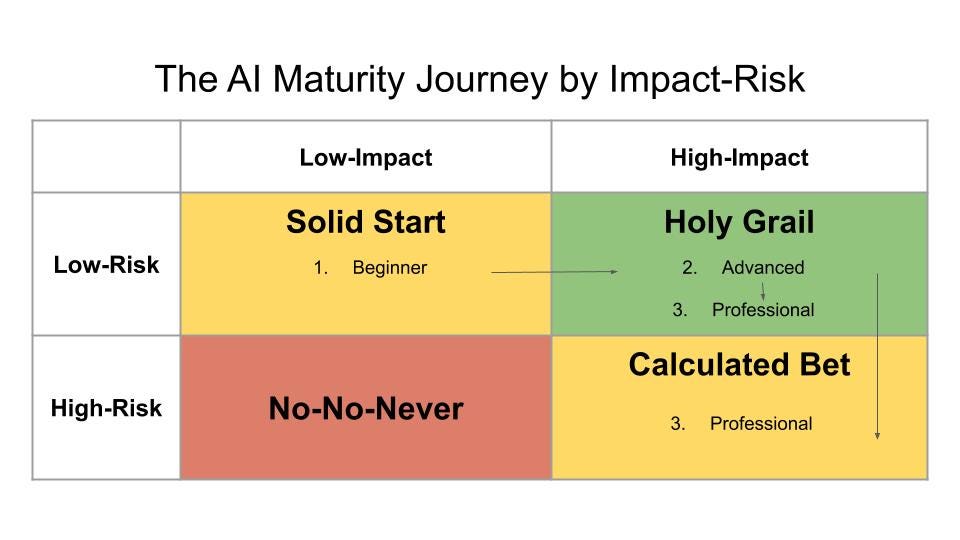
Porsche, for example, has emphasized AI as one of its focus topics to drive the future of its IT capabilities.
Tobias stresses the importance of understanding your companies position in the AI Maturity Journey. To him, high-impact, high-risk „Calculated Bets“ AI Projects are especially appealing when a company is looking for innovative new ways to solve existing problems. Innovative ideas are rarely of low-risk, yet are important to a company with an advanced level of AI Maturity. Google recommends a split of 70/20/10 of work on your core/feasible innovation/moonshot projects to continuously innovate.
Key Takeaways
When evaluating AI Projects, consider the Impact-Risk Matrix. Given your position along the AI Maturity Journey, focus on AI Projects from different categories.
- Impact = Addressable Audience * Opportunity
- Risk is best evaluated through the AI Project Canvas
- If you’re starting out with AI, look for low-impact, low-risk „Solid Start“ AI Projects to get started
- After your first learning’s, focus on high-impact, low-risk „Holy Grail“ AI Projects to drive value
- Once you have reached AI Maturity, allocate some resources on „Calculated Bet“ AI Projects from both high impact and risk categories
This post aims to give you a practitioner’s guide for evaluating different AI Projects. These methods have been tried and tested and are applied in the industry today.