The bigger model is not always the better model
Miniaturization of electronics started by NASA’s push became an entire consumer products industry. Now we’re carrying the complete works of Beethoven on a lapel pin listening to it in headphones. — Neil deGrasse Tyson, astrophysicist and science commentator
[…] the pervasiveness of ultra-low-power embedded devices, coupled with the introduction of embedded machine learning frameworks like TensorFlow Lite for Microcontrollers will enable the mass proliferation of AI-powered IoT devices. — Vijay Janapa Reddi, Associate Professor at Harvard University

This is the first in a series of articles on tiny machine learning. The goal of this article is to introduce the reader to the idea of tiny machine learning and its future potential. In-depth discussion of specific applications, implementations, and tutorials will follow in subsequent articles in the series.
Introduction
Over the past decade, we have witnessed the size of machine learning algorithms grow exponentially due to improvements in processor speeds and the advent of big data. Initially, models were small enough to run on local machines using one or more cores within the central processing unit (CPU).
Shortly after, computation using graphics processing units (GPUs) became necessary to handle larger datasets and became more readily available due to introduction of cloud-based services such as SaaS platforms (e.g., Google Colaboratory) and IaaS (e.g., Amazon EC2 Instances). At this time, algorithms could still be run on single machines.
More recently, we have seen the development of specialized application-specific integrated circuits (ASICs) and tensor processing units (TPUs), which can pack the power of ~8 GPUs. These devices have been augmented with the ability to distribute learning across multiple systems in an attempt to grow larger and larger models.
This came to a head recently with the release of the GPT-3 algorithm (released in May 2020), boasting a network architecture containing a staggering 175 billion neurons — more than double the number present in the human brain (~85 billion). This is more than 10x the number of neurons than the next-largest neural network ever created, Turing-NLG (released in February 2020, containing ~17.5 billion parameters). Some estimates claim that the model cost around $10 million dollars to train and used approximately 3 GWh of electricity (approximately the output of three nuclear power plants for an hour).
Tiny machine learning (tinyML) is the intersection of machine learning and embedded internet of things (IoT) devices. The field is an emerging engineering discipline that has the potential to revolutionize many industries.
Such improvements are also applicable to larger models, which may lead to efficiency increases in machine learning models by orders of magnitude with no impact on model accuracy. As an example, the Bonsai algorithm developed by Microsoft can be as small as 2 KB but can have even better performance than a typical 40 MB kNN algorithm, or a 4 MB neural network. This result may not sound important, but the same accuracy on a model 1/10,000th of the size is quite impressive. A model this small can be run on an Arduino Uno, which has 2 KB RAM available — in short, you can now build such a machine learning model on a $5 microcontroller.
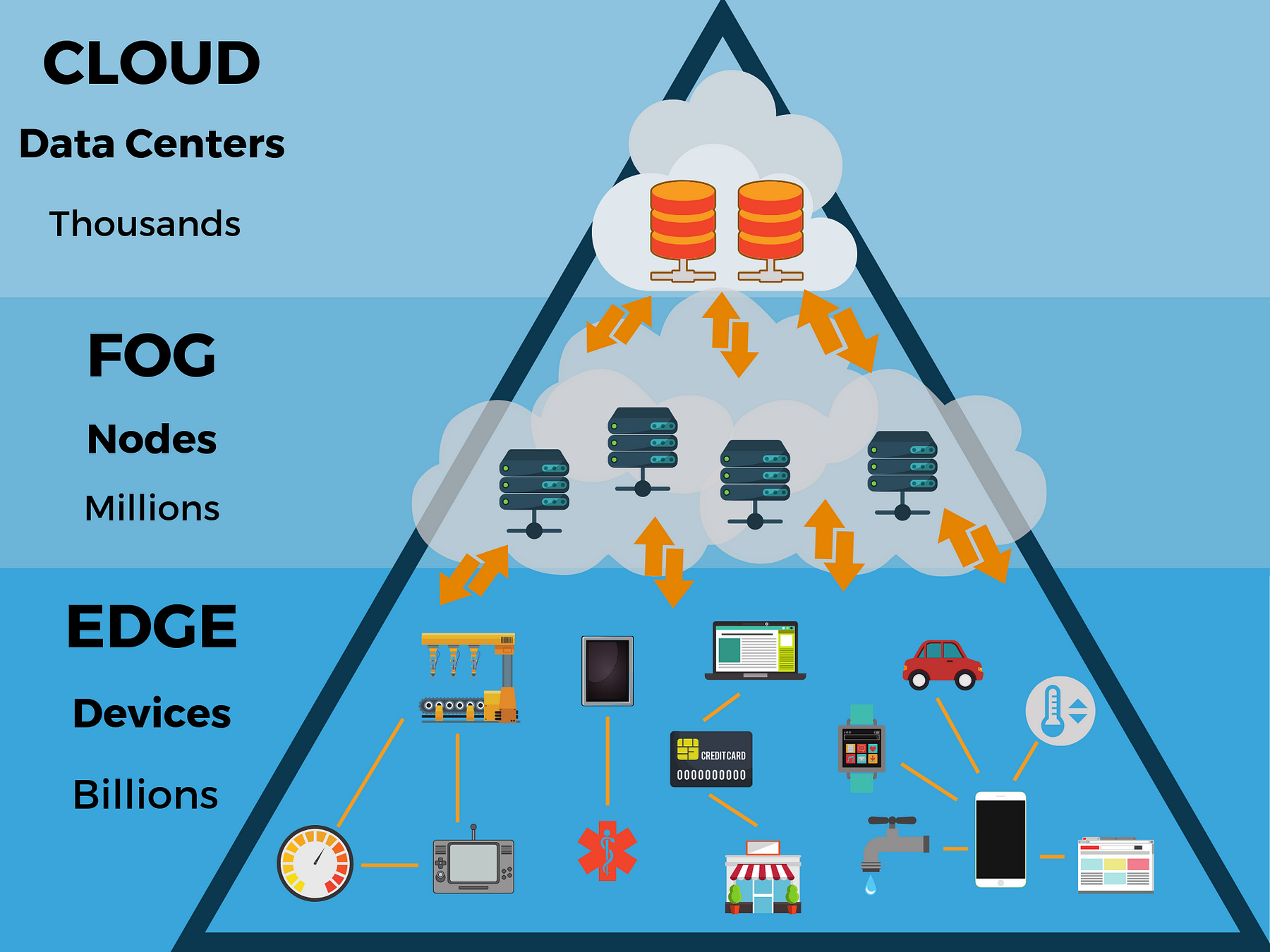
Examples of TinyML
Clearly, these are not the only possible applications of TinyML. In fact, TinyML presents many exciting opportunities for businesses and hobbyists alike to produce more intelligent IoT devices. In a world where data is becoming more and more important, the ability to distribute machine learning resources to memory-constrained devices in remote locations could have huge benefits on data-intensive industries such as farming, weather prediction, or seismology.
It is without a doubt that empowering edge devices with the capability of performing data-driven processing will produce a paradigm shift for industrial processes. As an example, devices that are able to monitor crops and send a “help” message when it detects characteristics such as soil moisture, specific gases (for example, apples emit ethane when ripe), or particular atmospheric conditions (e.g., high winds, low temperatures, or high humidity), would provide massive boosts to crop growth and hence crop yield.
As another example, a smart doorbell might be fitted with a camera that can use facial recognition to determine who is present. This could be used for security purposes, or even just so that the camera feed from the doorbell is fed to televisions in the house when someone is present so that the residents know who is at the door.
Two of the main focus areas of tinyML currently are:
Keyword spotting. Most people are already familiar with this application. “Hey Siri” and “Hey Google” are examples of keywords (often used synonymously with hotword or wake word). Such devices listen continuously to audio input from a microphone and are trained to only respond to specific sequences of sounds, which correspond with the learned keywords. These devices are simpler than automatic speech recognition (ASR) applications and utilize correspondingly fewer resources. Some devices, such as Google smartphones, utilize a cascade architecture to also provide speaker verification for security.
A more broad overview of current machine learning use cases of TinyML is shown below.
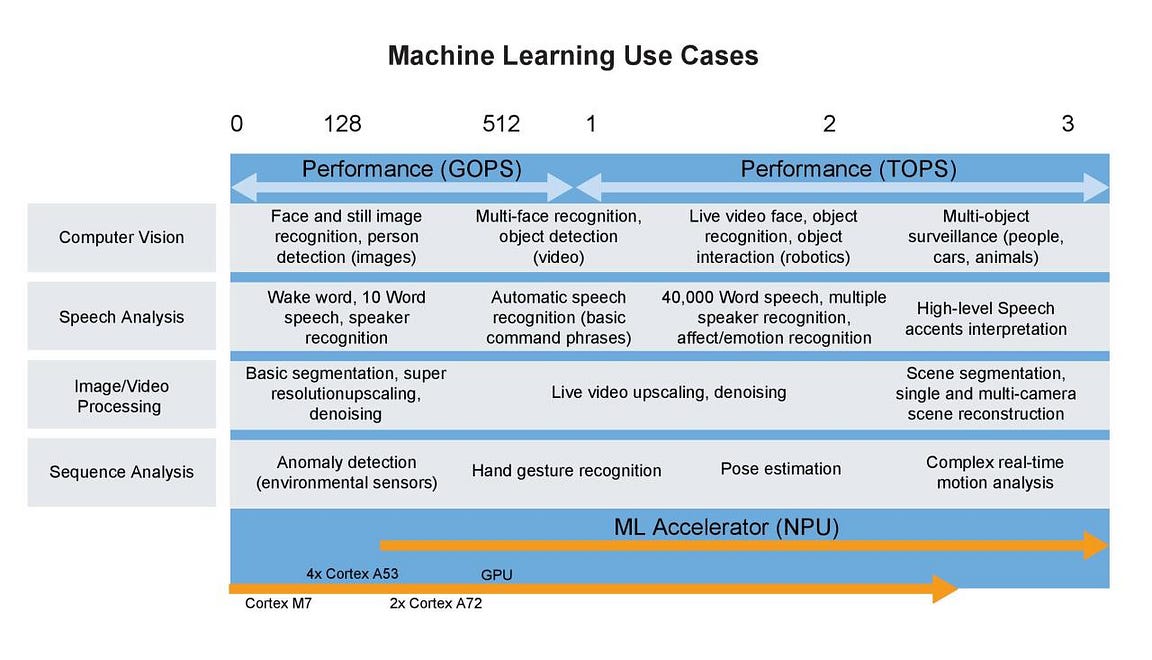
How TinyML Works

Model Distillation
The below diagram illustrates the process of knowledge distillation.
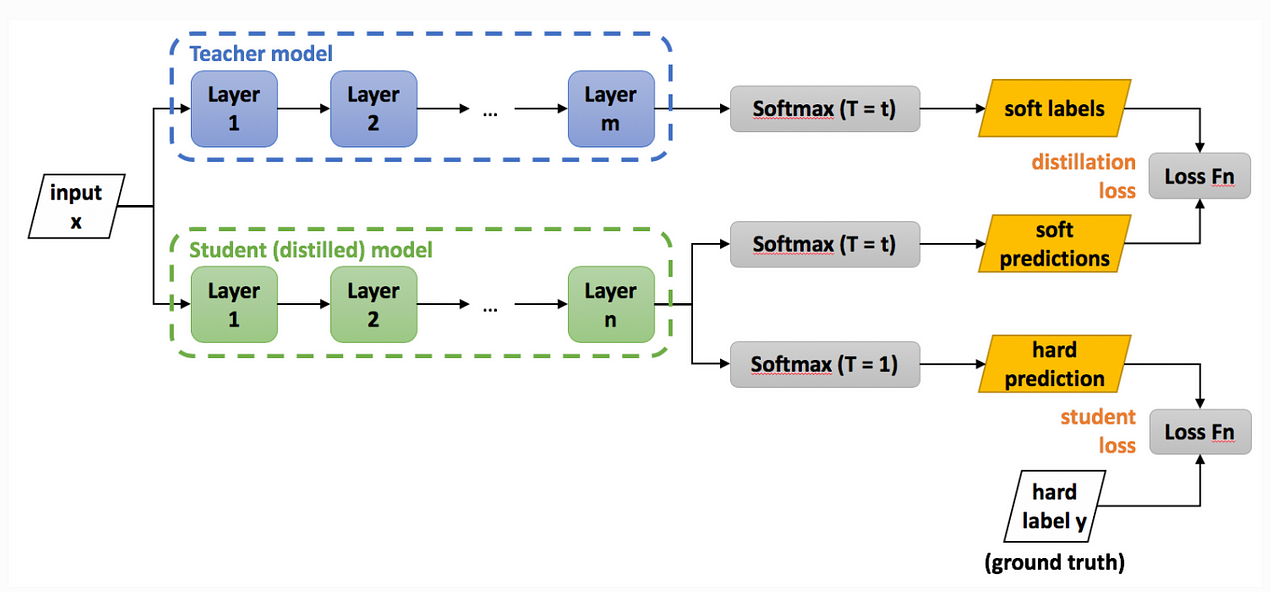

Quantization
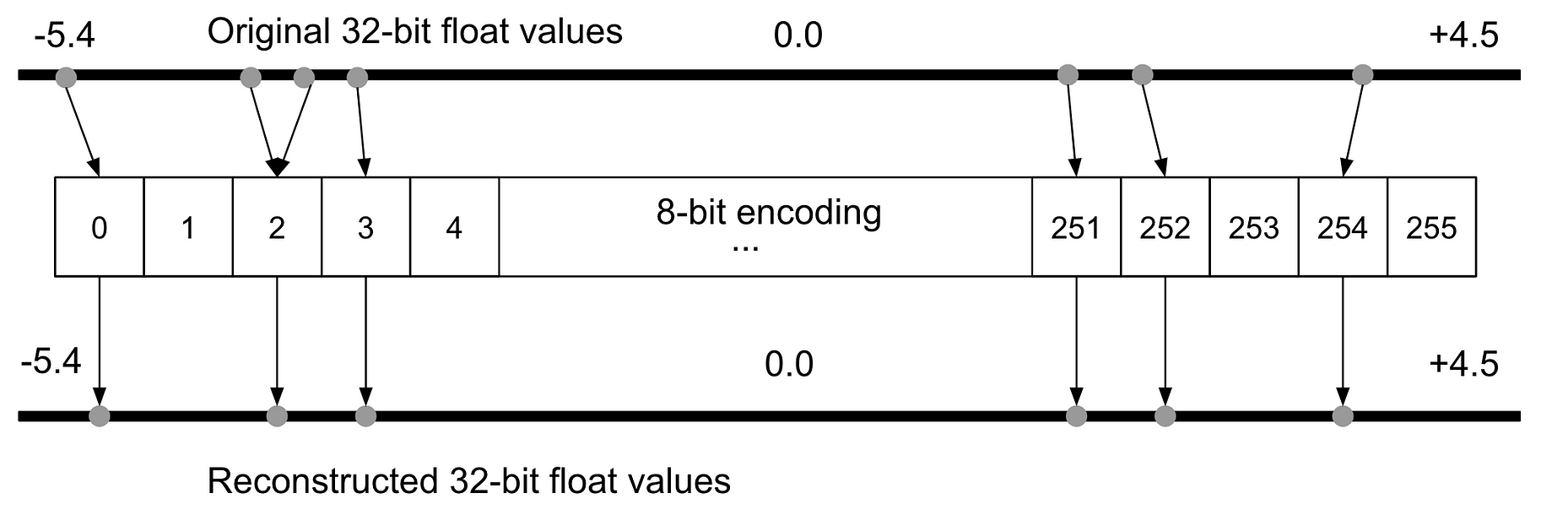
Huffman Encoding
Compilation
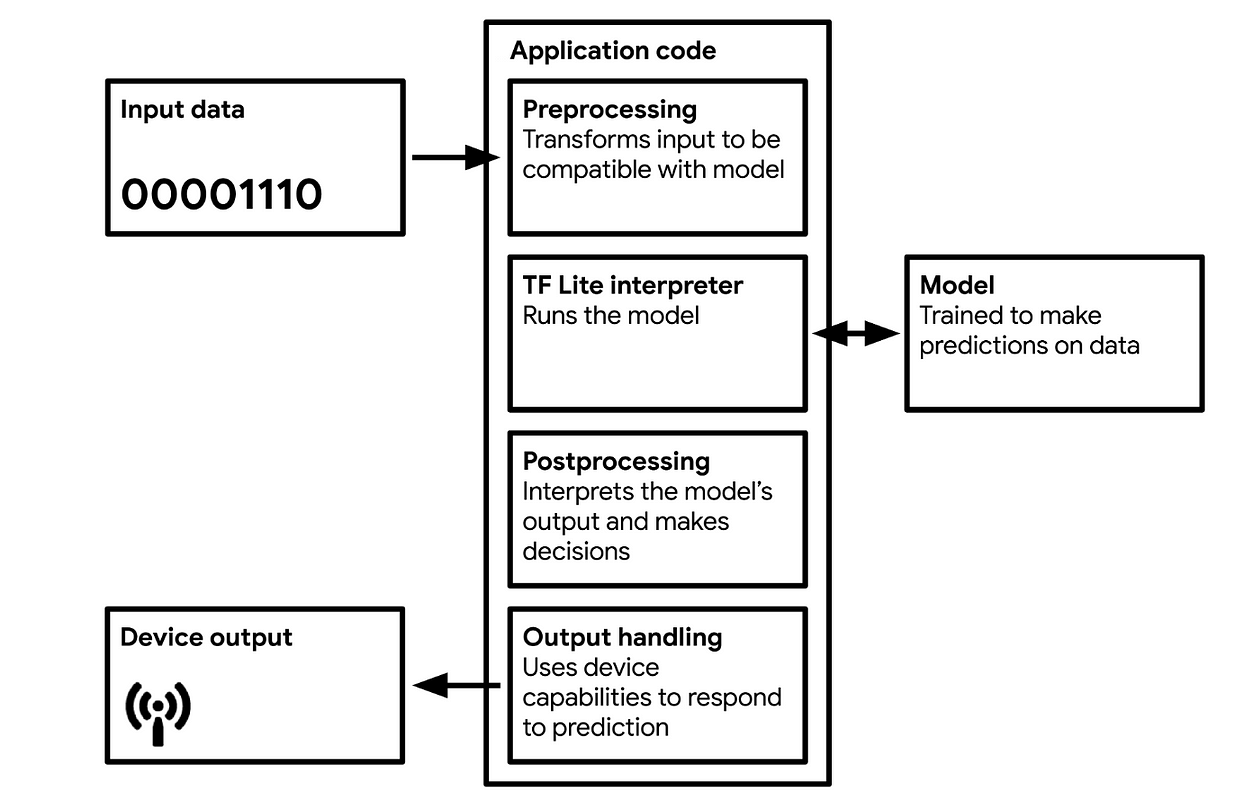
Why not train on-device?
Training on-device brings about additional complications. Due to reduced numerical precision, it becomes exceedingly difficult to guarantee the necessary level of accuracy to sufficiently train a network. Automatic differentiation methods on a standard desktop computer are approximately accurate to machine precision. Computing derivatives to the accuracy of 10^-16 is incredible, but utilizing automatic differentiation on 8-bit values will result in poor results. During backpropagation, these derivatives are compounded and eventually used to update neural parameters. With such a low numerical precision, the accuracy of such a model may be poor.
The first paper looking at reducing numerical precision in deep learning was the 2015 paper “Deep Learning with Limited Numerical Precision” by Suyog Gupta and colleagues. The results of this paper were interesting, showing that the 32-bit floating-point representation could be reduced to a 16-bit fixed-point representation with essentially no degradation in accuracy. However, this is the only case when stochastic roundingis used because, on average, it produces an unbiased result.
How about compute-efficiency?
Models can also be tailored to make them more compute-efficient. Model architectures widely deployed on mobile devices such as MobileNetV1 and MobileNetV2 are good examples. These are essentially convolutional neural networks that have recast the convolution operation to make it more compute-efficient. This more efficient form of convolution is known as depthwise separable convolution. Architectures can also be optimized for latency using hardware-based profiling and neural architecture search, which are not covered in this article.
The Next AI Revolution
References
That being said, neural networks have been trained using 16-bit and 8-bit floating-point numbers.